Deep Deformable 3D Caricatures with Learned Shape Control
Yucheol Jung, Wonjong Jang, Soongjin Kim Jiaolong Yang, Xin Tong, Seungyong Lee
SIGGRAPH 2022
[Paper] [ArXiv] [GitHub] [Supplementary Video] [Gallery (Including comparison with Alive Caricature)]
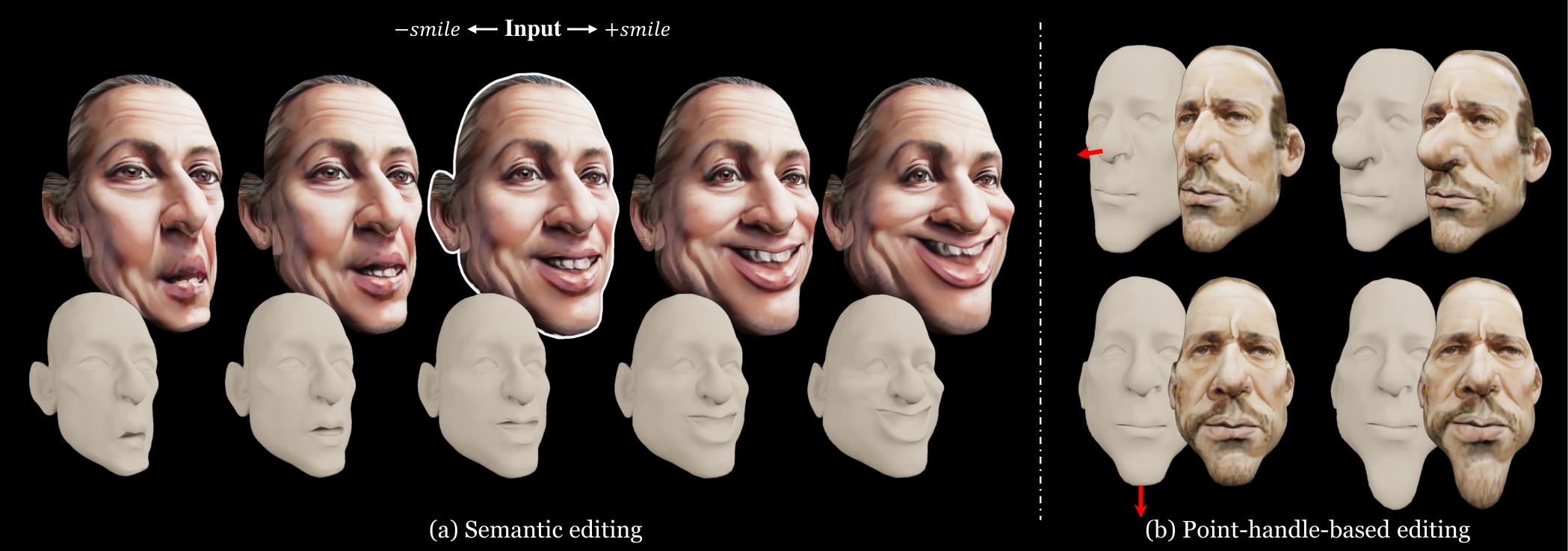
Abstract A 3D caricature is an exaggerated 3D depiction of a human face. The goal of this paper is to model the variations of 3D caricatures in a compact parameter space so that we can provide a useful data-driven toolkit for handling 3D caricature deformations. To achieve the goal, we propose an MLP-based framework for building a deformable surface model, which takes a latent code and produces a 3D surface. In the framework, a SIREN MLP models a function that takes a 3D position on a fixed template surface and returns a 3D displacement vector for the input position. We create variations of 3D surfaces by learning a hypernetwork that takes a latent code and produces the parameters of the MLP. Once learned, our deformable model provides a nice editing space for 3D caricatures, supporting label-based semantic editing and point-handle-based deformation, both of which produce highly exaggerated and natural 3D caricature shapes. We also demonstrate other applications of our deformable model, such as automatic 3D caricature creation.
Overview
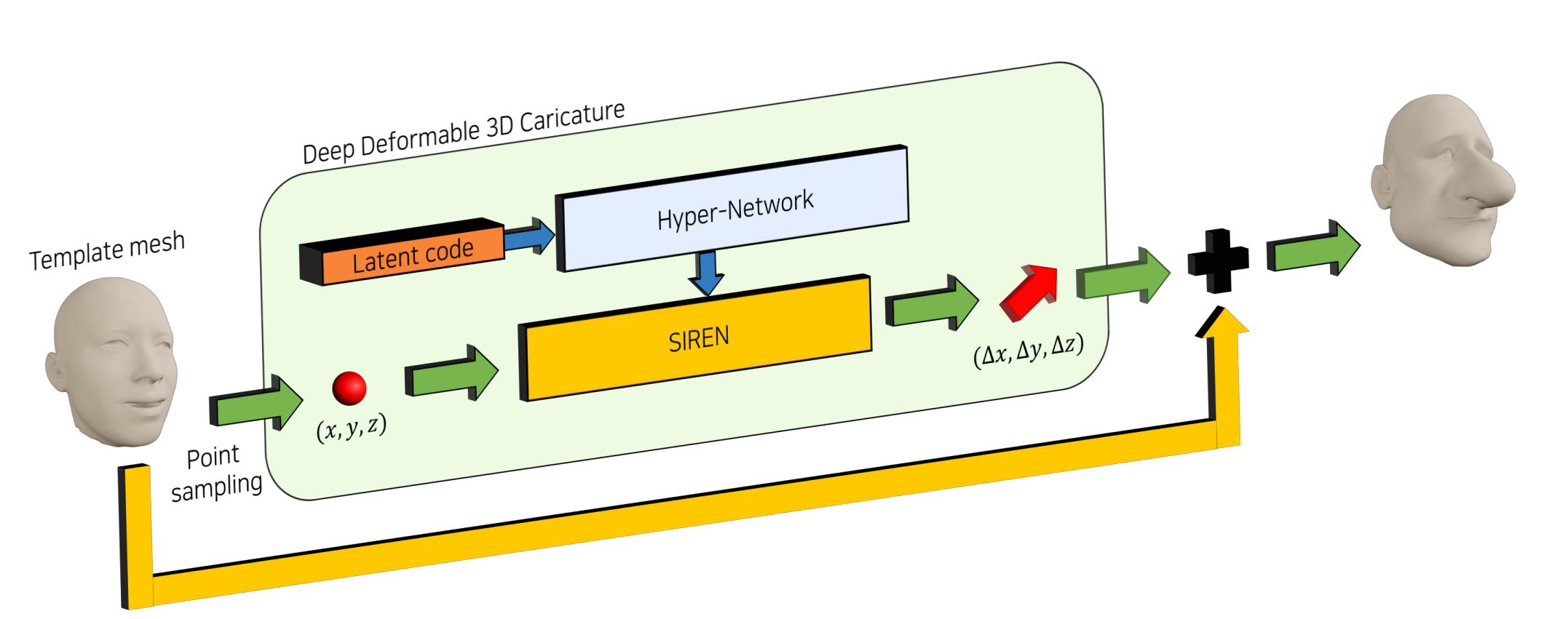
We adopt hyper-network architecture to model the latent space of highly complex 3D caricature shapes. Given a latent code, a SIREN MLP is generated. The SIREN MLP provides a mapping from a 3D coordinate on a fixed template mesh to a 3D displacement that is applied to the point. Once the model is trained, the learned mapping from a latent code to a 3D shape is used for various shape control.